Introduction
The study was conducted by Waterplan members (Hemant Servia, Frauke Albrecht, Samuel Saxe, Nicolas Bierti) and Suntory Global Innovation Center Ltd. Institute for Water Science members (Masatoshi Kawasaki and Shun Kurihara).
Predicting how much water flows in rivers without direct measurements has always been challenging, especially in areas where we can't easily access or monitor these rivers. The tools traditionally used to estimate this aren't always effective when applied to different locations, particularly outside of the United States. This limitation impedes informed water management decisions in areas with these rivers.
Recently, a breakthrough study in Japan by Waterplan and Suntory Institute for Water Science has begun to change this, achieving impressive results. An advanced technology called deep learning is being used —specifically a method known as long short-term memory (LSTM) networks.
This innovative approach goes beyond water measurement; it's about better informing our water management decisions and planning for environmental sustainability in regions where data is scarce. This research is a significant step forward in helping us understand and protect our water supplies on a global scale.
This study has been submitted and selected for oral presentation by the European Geosciences Union (EGU) for the 2024 conference in Vienna (session HS3.4 on April 19, 2024).
Enhancing River Flow Prediction with Deep Learning
Traditional hydrological models often struggle to predict river flows accurately beyond their calibrated areas. To overcome this limitation, this study employed advanced techniques called Long Short-Term Memory (LSTM) networks, a type of deep learning model. These networks are good at understanding how data changes over time and using these learnings to make predictions, such as predicting river flows that vary hourly, daily, or seasonally.
The study trained these models on multiple catchments in Japan using streamflow regionalization. By training LSTM models on diverse hydrometeorological data and catchment characteristics, the models gained insights that improved predictions not only within the training areas but also in other basins across the region.
Diverse Catchments in Japan Were Analyzed
The study analyzed 211 catchments across Japan's main islands: Kyushu, Shikoku, Honshu, and Hokkaido. These catchments represented a diverse range of hydrological systems, covering over 43% of Japan's total land area.
After thorough data cleaning and refinement, 198 catchments were divided into training, validation, and holdout test sets. The models were provided with daily inputs of dynamic and static variables, with a focus on dynamic features to improve predictive accuracy.
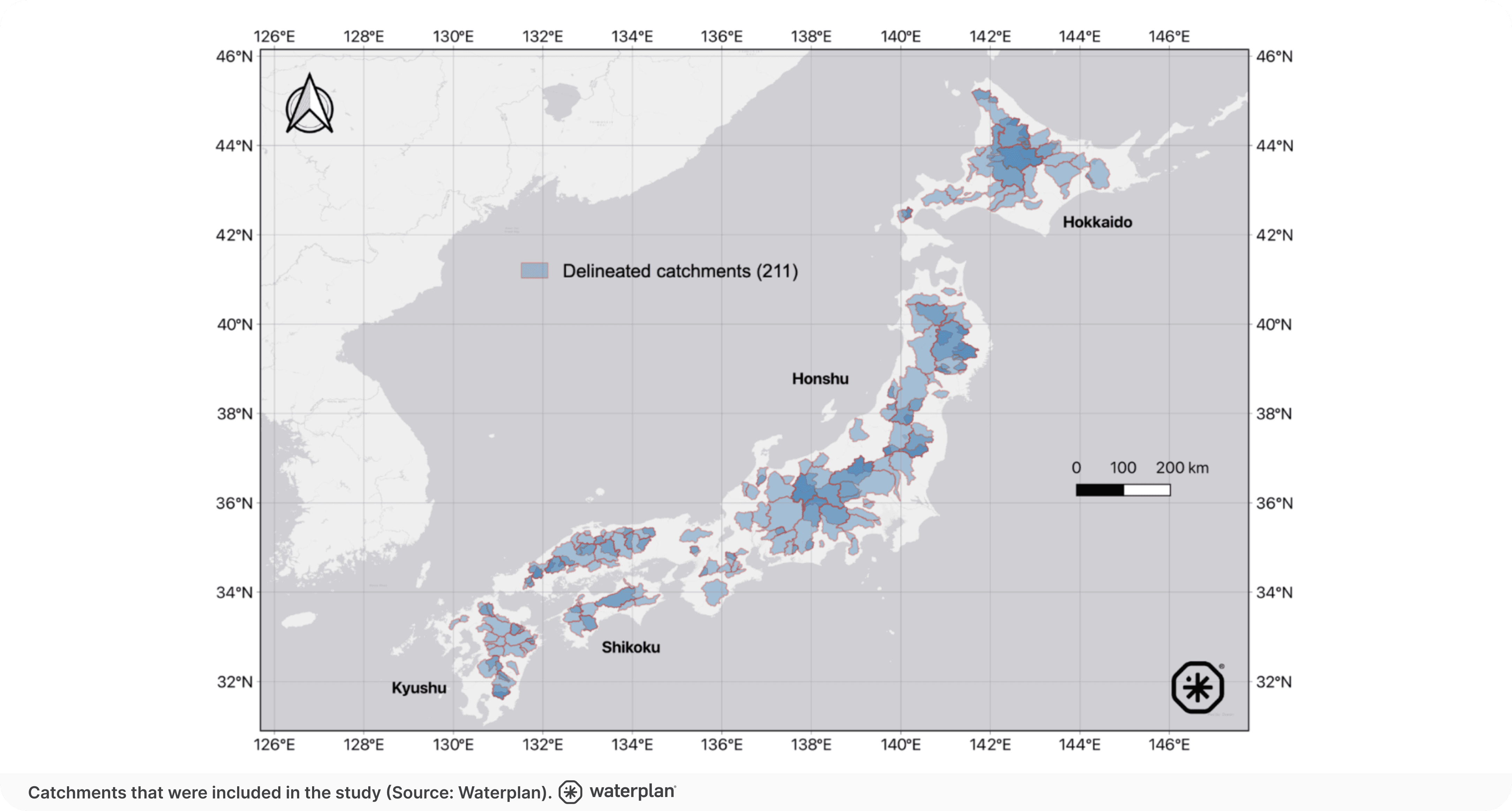
The Model Had Impressive Results
The final model exhibited remarkable performance metrics.
Specifically, the model had a median Nash-Sutcliffe Efficiency (NSE) of 0.96, 0.83, and 0.78, along with a median correlation coefficient of 0.98, 0.92, and 0.91 for training, validation, and test catchments, respectively. Notably, 90% of validation catchments achieved NSE values above 0.50, and 97% showed correlations exceeding 0.70. Similarly, 77% and 91% of test catchments met these thresholds, underscoring the model's robustness and generalization ability.
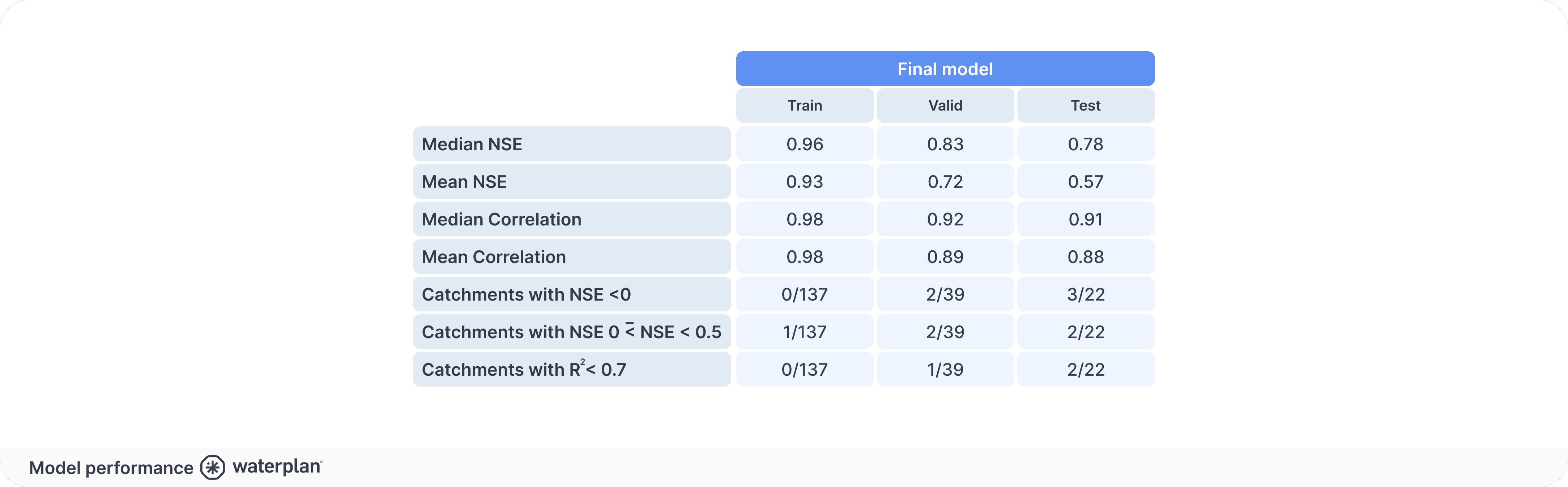
Better Water Data for Informed Water Management Decisions
In conclusion, this research in Japan marks a major step forward in hydrological modeling. By challenging traditional models and adopting innovative approaches, it sets the stage for more precise and reliable global hydrological predictions. This advancement can significantly enhance water data availability and accuracy, leading to more informed water management decisions.
It’s also worth mentioning that Waterplan is already involved in similar projects worldwide, such as in Spain and the USA, promising advancements in hydrological science globally.
You can learn more about water risk and the importance of having reliable data in this article.
ブログ
Get insights, expert analysis and tips on measuring, reporting, and responding to water risk